Lucas Lopes' PhD Defence
Date and Time
Location
Room 141 and Teams: https://teams.microsoft.com/l/meetup-join/19%3ameeting_NTJhYWIzMDMtNmU3ZS00OWViLTg3NTYtZThmOTZiYTViYjA1%40thread.v2/0?context=%7b%22Tid%22%3a%22be62a12b-2cad-49a1-a5fa-85f4f3156a7d%22%2c%22Oid%22%3a%22fbd28915-dda5-478f-8ecb-a3682dcf0c3a%22%7d
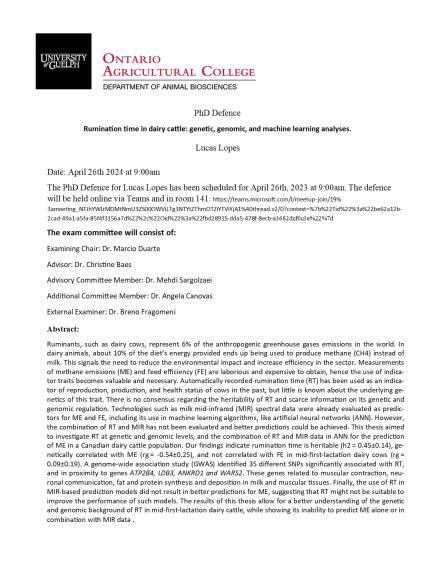
Details
Rumination time in dairy cattle: genetic, genomic, and machine learning analyses.
Ruminants, such as dairy cows, represent 6% of the anthropogenic greenhouse gases emissions in the world. In dairy animals, about 10% of the diet’s energy provided ends up being used to produce methane (CH4) instead of milk. This signals the need to reduce the environmental impact and increase efficiency in the sector. Measurements of methane emissions (ME) and feed efficiency (FE) are laborious and expensive to obtain, hence the use of indicator traits becomes valuable and necessary. Automatically recorded rumination time (RT) has been used as an indicator of reproduction, production, and health status of cows in the past, but little is known about the underlying genetics of this trait. There is no consensus regarding the heritability of RT and scarce information on its genetic and genomic regulation. Technologies such as milk mid-infrared (MIR) spectral data were already evaluated as predictors for ME and FE, including its use in machine learning algorithms, like artificial neural networks (ANN). However, the combination of RT and MIR has not been evaluated and better predictions could be achieved. This thesis aimed to investigate RT at genetic and genomic levels, and the combination of RT and MIR data in ANN for the prediction of ME in a Canadian dairy cattle population. Our findings indicate rumination time is heritable (h2 = 0.45±0.14), genetically correlated with ME (rg = -0.54±0.25), and not correlated with FE in mid-first-lactation dairy cows (rg = 0.09±0.19). A genome-wide association study (GWAS) identified 35 different SNPs significantly associated with RT, and in proximity to genes ATP2B4, LDB3, ANKRD1 and WARS2. These genes related to muscular contraction, neuronal communication, fat and protein synthesis and deposition in milk and muscular tissues. Finally, the use of RT in MIR-based prediction models did not result in better predictions for ME, suggesting that RT might not be suitable to improve the performance of such models. The results of this thesis allow for a better understanding of the genetic and genomic background of RT in mid-first-lactation dairy cattle, while showing its inability to predict ME alone or in combination with MIR data.