Elena Wang's MSc Defence
Date and Time
Location
Teams: https://teams.microsoft.com/l/meetup-join/19%3ameeting_YTkyMDBlNjItNWY2Ny00OWE4LWJlYzUtYTI3YThhMzJjZTk2%40thread.v2/0?context=%7b%22Tid%22%3a%22be62a12b-2cad-49a1-a5fa-85f4f3156a7d%22%2c%22Oid%22%3a%22fbd28915-dda5-478f-8ecb-a3682dcf0c3a%22%7d
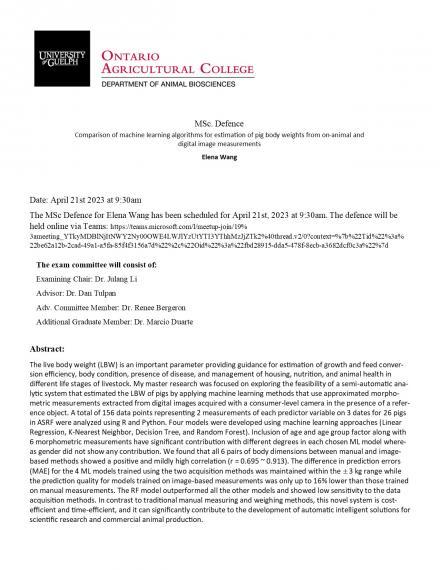
Details
The live body weight (LBW) is an important parameter providing guidance for estimation of growth and feed conversion efficiency, body condition, presence of disease, and management of housing, nutrition, and animal health in different life stages of livestock. My master research was focused on exploring the feasibility of a semi-automatic analytic system that estimated the LBW of pigs by applying machine learning methods that use approximated morphometric measurements extracted from digital images acquired with a consumer-level camera in the presence of a reference object. A total of 156 data points representing 2 measurements of each predictor variable on 3 dates for 26 pigs in ASRF were analyzed using R and Python. Four models were developed using machine learning approaches (Linear Regression, K-Nearest Neighbor, Decision Tree, and Random Forest). Inclusion of age and age group factor along with 6 morphometric measurements have significant contribution with different degrees in each chosen ML model whereas gender did not show any contribution. We found that all 6 pairs of body dimensions between manual and image-based methods showed a positive and mildly high correlation (r = 0.695 ~ 0.913). The difference in prediction errors (MAE) for the 4 ML models trained using the two acquisition methods was maintained within the ± 3 kg range while the prediction quality for models trained on image-based measurements was only up to 16% lower than those trained on manual measurements. The RF model outperformed all the other models and showed low sensitivity to the data acquisition methods. In contrast to traditional manual measuring and weighing methods, this novel system is cost-efficient and time-efficient, and it can significantly contribute to the development of automatic intelligent solutions for scientific research and commercial animal production.